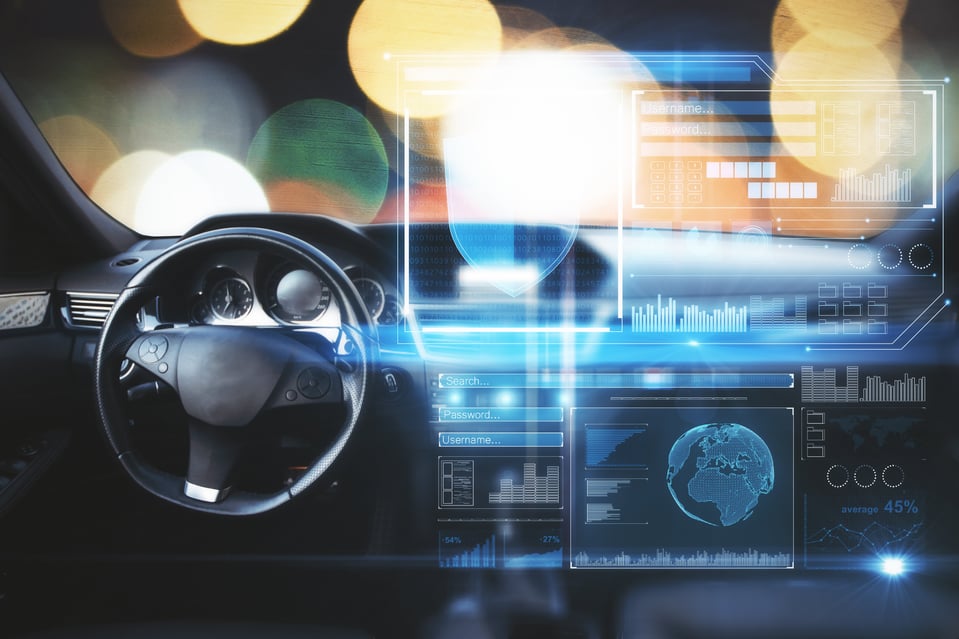
Blogs
Image Quality Testing for AR-HUD Performance
An automotive head-up display system, or HUD, aims to improve passenger safety by projecting information such as navigation, warnings, and points of interest, to the driver’s visual field, ideally in real-time. The intention is to keep the driver’s focus on the road by reducing the eyes-off-the-road time and increasing situational awareness. Conventional HUDs produce a 2D image with a fixed, or static, projection. However, Augmented Reality (AR) HUDs produce a 3D image that adds depth and curvature to conventional HUD imagery and features a variable, or dynamic, projection.
Conventional 2D HUD offers a static projection. 3D AR-HUD offers depth and curvature with dynamic projection, but the large field of view (FOV), image distance, and dynamic nature present unique optical metrology challenges.
AR-HUD is a new technology that, as of 2021, is only available in limited premium car models. AR-HUD delivers a 3D image that offers various information embedded in the real-world view. In an ideal HUD, the field of view (FOV) should be quite large, the virtual image should be at a distance longer than 10 meters, and the system should continuously integrate information from the vehicle's interior and exterior surroundings.
There are different technologies to build head-up display optics. As in see-through AR applications, the most common display or light engine technologies are DLP (Digital Light Processor), and LBS (Laser Beam Scanning). 3D Computational Holography is another technology that shows promise for AR-HUDs. Testing the performance of AR-HUD technologies requires a suite of optical metrology toolsets for a fast, accurate, automated way to achieve performance and production goals.
Diffractive Optics
Since both optics and display-related technologies are relatively new, there are known challenges with image quality depending on the selected display and optics technology. Issues include:
- Distortions
- Alignment performance of the projected 3D objects in real-world in the eye-box
- Variable virtual image distance
Diffractive optical elements substantially increase virtual image size and project to infinite or multiple focal distances, without changing the volume of the AR-HUD unit, according to Nokia Bell Labs.
The use of diffractive optics may solve significant performance barriers but present quality metrology challenges such as the measurement of waveguide performance (efficiency, uniformity, contrast, modulation transfer function, etc.).
Validation of Optical Performance
As in any AR-based solution, an ideal optical metrology requires mimicking the human eye with a single shot capture of the entire FOV that also includes the color information. Some of the test metrics which are used for validating the optical performance of AR-HUDs are defined under IEC 63145, the international standard for measurement conditions and methods for eyewear displays.
The most common test metrics for evaluating and validating the optical performance of AR-HUDs are:
Virtual Image Distance (VID)
AR-HUDs can have up to 20 meters of VID. A long VID, like that of an AR-based setup, object tracking is easier due to the working principles of the human eye. The human eye adjusts the focus less frequently when the objects are farther, which reduces eye fatigue and maintains driver focus.
During measurement of the VID, a slanted edge square pattern or Michelson contrast line pattern is imaged and ESF (edge spread function per ISO 12233) is super-sampled based on the offset caused by the 5-degree angle of the edge.
To assess the optical performance of the AR-HUDs, traditional test metrics are also used.
Image Skew
This term describes any optical path that is not in a plane of symmetry. In FOV measurement, the image skew is calculated from the image’s corner coordinates.
Image Flatness
In measuring the VID, Michelson contrast is calculated over the selected FOV for selected points within the FOV. Then, the VID differences between the selected points are reported as the image flatness.
Ghost and Flare
To measure ghost and flare, a selected target pattern is imaged over the selected focus distance range within the eye-box and using the selected exposure range. In gradually increasing the exposure range, real images become overexposed, and artifacts become more visible.
Gamma
Gamma is an important performance measurement — it is the luminance linearity that shows how smooth black transitions to white on a digital display. To measure Gamma, different numbers of samples are used to measure the luminance required to create the curve.
Lateral Chromatic Aberration (LCA)
This is analyzed by implementing a set of black dot grid patterns that has a similar use with radial chromatic displacement measurements. During the measurement, for each color channel (RGB) individual images are taken. Then, as in distortion analysis, the center points of each color are compared. In some cases, LCA measurements help evaluate the individual VID for each color channel separately.
Additional tests are usually performed to validate AR-HUD image quality such as:
- FOV Analysis
- Eye-Box Size Analysis
- Geometric Distortion Analysis
- Contrast Ratio Analysis
- Luminance Non-Uniformity Analysis
- Color Analysis
- Color Non-Uniformity Analysis
Advanced test cases simulating different driving scenarios like day, night, high exposure of sunshine, low and high temperature, or under various car headlight exposures, etc. are also performed to validate the image quality and optical performance of AR-HUDs.
Figure 1: AR-HUD Image Quality Tester Concept Picture
With more than 10 years of optical metrology expertise in diffractive optics and other AR display technologies, OptoFidelity provides proof of concept studies and fully automated robotic systems for validating optical characteristics as well as overall system functionality and performance of AR-HUDs. Request a consultation to discuss your testing requirements.
Written by